
Canadian
Mathematical
Society
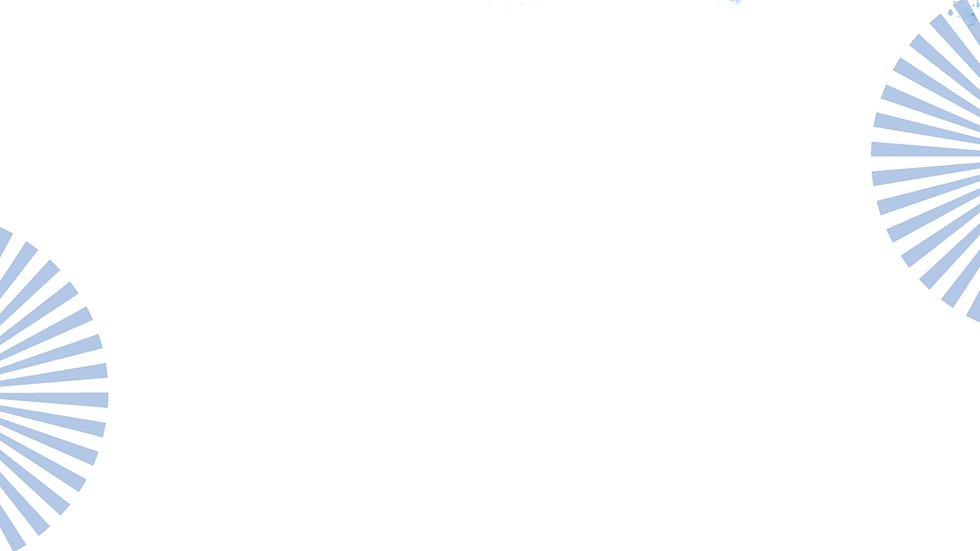
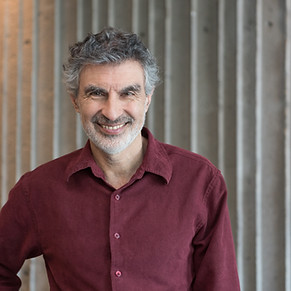
Yoshua Bengio
Université de Montréal, and the Founder and Scientific Director of Mila – Quebec AI Institute
Yoshua Bengio is Full Professor in the Department of Computer Science and Operations Research at Université de Montreal, as well as the Founder and Scientific Director of Mila and the Scientific Director of IVADO. Considered one of the world’s leaders in artificial intelligence and deep learning, he is the recipient of the 2018 A.M. Turing Award with Geoff Hinton and Yann LeCun, known as the Nobel prize of computing.
​
He is a Fellow of both the Royal Society of London and Canada, an Officer of the Order of Canada, Knight of the Legion of Honor of France, and a Canada CIFAR AI Chair.
Welcome and Opening Remarks at 4:45 pm
Public Lecture: 5:00 pm - 6:00 pm
Recognized worldwide as one of the leading experts in artificial intelligence, Yoshua Bengio is most known for his pioneering work in deep learning, earning him the 2018 A.M. Turing Award, “the Nobel Prize of Computing,” with Geoffrey Hinton and Yann LeCun. He is Full Professor at Université de Montréal, and the Founder and Scientific Director of Mila – Quebec AI Institute. He co-directs the CIFAR Learning in Machines & Brains program as Senior Fellow and acts as Scientific Director of IVADO. In 2019, he was awarded the prestigious Killam Prize and in 2022, became the most cited computer scientist in the world. He is a Fellow of both the Royal Society of London and Canada, Knight of the Legion of Honor of France, Officer of the Order of Canada, and a Canada CIFAR AI Chair. Concerned about the social impact of AI, he actively contributed to the Montreal Declaration for the Responsible Development of Artificial Intelligence.
Abstract
Mathematical challenges towards safe AI
Advances in algorithms and computational capabilities of AI systems based on deep learning have been impressive and herald possibly disruptive transformations in coming years and decades, with great potential for both benefits and risks for humanity. The three winners of the Turing award for deep learning (2018) expect that broad human-level capabilities are likely to be achieved within just a few years or decades and industry is investing billions of dollars per month which are likely to accelerate this process. However, we do not yet know how to design provably safe and controllable AI systems, i.e., systems that behave as we intend. This misalignment could threaten democracy, national security and possibly our collective future either due to malicious actors or a loss of control to runaway AIs. Worse, arguments have been made suggesting that the state-of-the-art AI methodology, based on reinforcement learning, would yield less and less safety as computational power increases. This presentation will argue that there may be a way to design AI systems with probabilistic safety guarantees that improve as we increase the computational capabilities of the underlying neural networks. This would rely on efficient and amortized Bayesian inference in learned causal models, designing AI systems inspired by how scientists and mathematicians come up with theories that are compatible with reason and observed evidence.